Strengthening AI Security in India: Key Capabilities and Approaches
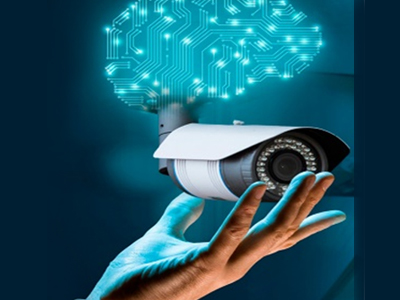
As Artificial Intelligence (AI) continues to revolutionize industries across the globe, India stands at the forefront of this technological transformation. From healthcare to finance, manufacturing to agriculture, AI is being leveraged to drive efficiency, innovation, and growth. However, the rapid adoption of AI also brings significant security challenges. As organizations in India embark on their AI journeys, ensuring the security of AI systems and data has become paramount. This article explores the new capabilities and strategies that can help secure AI transformation in India.
The Growing Importance of AI in India
AI is no longer just a futuristic concept; it is a reality that is transforming the way businesses operate in India. The Indian government has recognized AI as a key driver of economic growth and has launched various initiatives to promote its development and adoption. According to a report by NASSCOM, AI could contribute $450-500 billion to India’s GDP by 2025, representing 10% of the country’s GDP. This potential is being harnessed across various sectors:
• Healthcare: AI is being used to improve diagnostics, personalized treatment, and drug discovery.
• Finance: AI-driven algorithms are enhancing fraud detection, credit scoring, and customer service.
• Agriculture: AI is helping farmers with predictive analytics for crop yields and weather patterns.
• Manufacturing: AI is optimizing supply chains, predictive maintenance, and quality control.
While AI offers tremendous benefits, it also poses unique security risks that need to be addressed to ensure the safe and ethical deployment of AI technologies.
Security Challenges in AI Transformation
The integration of AI into business processes introduces several security challenges that are different from traditional IT security issues. These challenges include:
1. Data Privacy and Protection: AI systems require vast amounts of data to function effectively. In India, where data privacy is a growing concern, ensuring the protection of sensitive information used by AI systems is critical. The implementation of the Personal Data Protection Bill (PDPB) will have significant implications for how AI systems handle personal data.
2. Algorithmic Bias: AI systems are only as good as the data they are trained on. Biased data can lead to biased algorithms, resulting in unfair or unethical outcomes. For instance, biased AI in hiring processes could discriminate against certain groups, leading to reputational and legal risks.
3. Adversarial Attacks: AI systems are vulnerable to adversarial attacks, where malicious actors manipulate input data to deceive the AI model. These attacks can lead to incorrect predictions or classifications, undermining the reliability of AI systems.
4. Explainability and Transparency: AI models, especially deep learning models, are often considered "black boxes" due to their complexity. This lack of transparency can be problematic in sectors like finance and healthcare, where understanding the reasoning behind AI decisions is crucial.
5. Compliance and Ethical Concerns: As AI becomes more integrated into critical decision-making processes, ensuring compliance with regulations and ethical standards becomes increasingly important. Organizations must navigate the complexities of AI ethics, including issues related to accountability, fairness, and human oversight.
New Capabilities to Secure AI Transformation
To address these challenges, several new capabilities and best practices have emerged to help organizations in India secure their AI transformation. These capabilities focus on enhancing data security, improving algorithmic integrity, and ensuring compliance with regulatory and ethical standards.
1. Enhanced Data Encryption and Privacy-Preserving AI
As data privacy becomes a focal point in AI, organizations are adopting advanced encryption techniques to secure data at rest and in transit. Homomorphic encryption and secure multi-party computation (SMPC) are two emerging technologies that allow AI models to perform computations on encrypted data without ever decrypting it. This ensures that sensitive information remains protected throughout the AI lifecycle.
Privacy-preserving AI techniques, such as federated learning, are also gaining traction. Federated learning enables AI models to be trained across multiple decentralized devices or servers without sharing raw data. This approach minimizes data exposure and helps organizations comply with data privacy regulations like the PDPB.
2. Bias Detection and Mitigation Tools
To address the issue of algorithmic bias, new tools and frameworks are being developed to detect and mitigate bias in AI models. Fairness-aware machine learning algorithms can identify biased patterns in training data and adjust the model to produce more equitable outcomes. Additionally, open-source libraries like IBM’s AI Fairness 360 and Microsoft’s Fairlearn provide organizations with tools to assess and improve the fairness of their AI systems.
These tools are essential for ensuring that AI systems operate ethically and do not perpetuate existing inequalities. By proactively addressing bias, organizations can reduce the risk of reputational damage and legal challenges.
3. Robust Adversarial Defenses
Adversarial attacks are a growing threat to AI systems, particularly in critical applications like autonomous vehicles and cybersecurity. To counter these threats, researchers are developing robust adversarial defenses. Techniques such as adversarial training, where AI models are trained on adversarial examples, can improve the resilience of AI systems against such attacks.
Moreover, organizations are increasingly adopting AI monitoring and anomaly detection systems to identify and respond to adversarial threats in real-time. These systems can detect unusual patterns in AI input data and trigger alerts or corrective actions to prevent malicious exploitation.
4. Explainable AI (XAI) Techniques
The need for transparency in AI decision-making has led to the development of explainable AI (XAI) techniques. XAI aims to make AI models more interpretable by providing insights into how they arrive at their decisions. Techniques like SHAP (Shapley Additive Explanations) and LIME (Local Interpretable Model-agnostic Explanations) are being used to generate explanations for complex models.
In India, where AI is being deployed in sensitive sectors like healthcare and finance, explainability is crucial for building trust and ensuring compliance with regulatory requirements. By adopting XAI techniques, organizations can provide stakeholders with the transparency needed to justify AI-driven decisions.
5. AI Governance and Ethical Frameworks
As AI becomes more pervasive, organizations are recognizing the need for robust AI governance and ethical frameworks. These frameworks provide guidelines for the responsible development and deployment of AI systems, ensuring that they align with organizational values and regulatory requirements.
In India, the NITI Aayog has proposed an AI strategy that emphasizes the importance of ethics, transparency, and accountability in AI development. Organizations are encouraged to establish AI ethics committees and implement AI governance policies that address issues such as data privacy, bias, and accountability. By doing so, they can mitigate risks and ensure that their AI initiatives are conducted responsibly.
6. Collaboration with AI Security Experts
Given the complexity of securing AI systems, organizations are increasingly collaborating with AI security experts and consultants. These experts can provide specialized knowledge in areas such as adversarial machine learning, data privacy, and AI ethics. Collaboration with academic institutions and participation in AI security research initiatives can also help organizations stay ahead of emerging threats and adopt best practices.
Furthermore, industry collaborations and partnerships can facilitate the sharing of knowledge and resources, enabling organizations to build more secure AI systems. In India, where AI is rapidly evolving, such collaborations are essential for fostering innovation while maintaining security.
Conclusion
As AI continues to transform industries in India, securing AI systems and data has become a critical priority. The challenges associated with AI transformation—such as data privacy, algorithmic bias, adversarial attacks, and compliance—require new capabilities and approaches to address them effectively.
By adopting advanced encryption techniques, bias detection tools, adversarial defenses, explainable AI, and robust governance frameworks, organizations in India can secure their AI initiatives and ensure that they are both effective and ethical. Collaboration with AI security experts and participation in industry research will further enhance the security of AI systems, paving the way for a secure and responsible AI transformation in India.